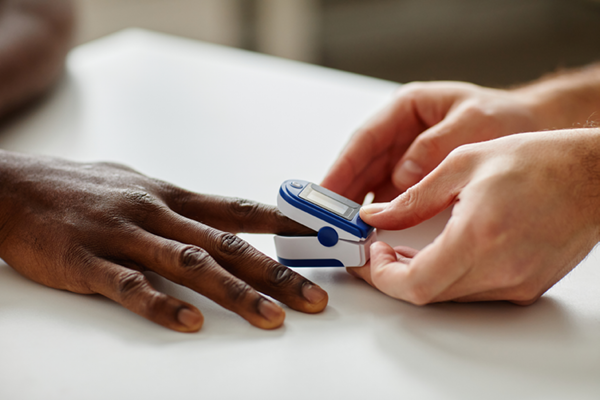
Pulse oximetry, a non-invasive method clinicians rely on to rapidly assess a patient's oxygen saturation, has been a standard of care for decades. However, mounting evidence shows that this technology is prone to inaccuracies in patients with darker skin tones, leading to hidden hypoxemia—a serious condition in which pulse oximetry readings falsely indicate adequate oxygen levels. Recent findings highlight that hidden hypoxemia disproportionately impacts patients with darker skin tones, increasing their risk of severe organ dysfunction and even death.
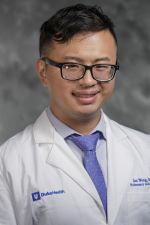
Responding to this critical issue, Dr. A. Ian Wong, assistant professor in the Division of Pulmonary, Allergy, and Critical Care Medicine and the Department of Biostatistics and Bioinformatics, is leading a five-year, $3.4 million NIH-funded research initiative aimed at improving clinical detection and management of hidden hypoxemia. The project, in collaboration with researchers from Emory University, leverages advanced machine learning techniques to identify patients at the highest risk using data already present in electronic health records (EHR).
The impetus for Dr. Wong’s research began shortly after a seminal 2021 publication drew attention to racial disparities in pulse oximetry accuracy. At the time, Dr. Wong, a clinician-researcher with a longstanding interest in pulse oximetry dating back to his biomedical engineering roots, recognized an urgent clinical need.
"Pulse oximeters were originally developed to signal severe hypoxemia, but they've become routine monitoring devices," Dr. Wong explained. "When you rely heavily on them for critical clinical decisions, even small inaccuracies can lead to significant harm, particularly in vulnerable patient populations."
Dr. Wong’s prior research, published in JAMA Open, demonstrated that hidden hypoxemia is not just a theoretical concern—it’s associated with a 41% increase in patient mortality and a substantial rise in organ dysfunction markers. These findings intensified the urgency to address the issue directly.
While some experts initially advocated for replacing existing pulse oximetry hardware, Dr. Wong’s experience indicated that this was neither realistic nor financially viable.
"Replacing every pulse oximeter device at Duke alone would cost tens of millions of dollars and nationwide could approach billions," Dr. Wong noted. "Most hospitals will wait for equipment to reach end-of-life before investing in new technologies, potentially taking decades to achieve widespread change."
Instead, Dr. Wong’s research focuses on developing pragmatic solutions that can be deployed immediately. By analyzing subtle but clinically significant signals from routinely collected EHR data—including laboratory markers indicative of organ dysfunction—the research team aims to build predictive models that can identify patients with hidden hypoxemia in real-time. These models could then prompt clinicians to order arterial blood gas (ABG) tests, considered the gold standard for oxygen measurement, or initiate more aggressive oxygen therapy, potentially averting serious health complications.
A key innovative component of Dr. Wong’s research is the incorporation of skin tone measurements into predictive models. Recognizing the inadequacy of racial categories as proxies for skin pigmentation, the team is evaluating multiple objective measurement methods, including colorimeters, spectrophotometers, visual scales, and even mobile phone camera technology. This comprehensive approach aims to determine the most accurate and practical method to characterize skin tone in critically ill patients.
"Skin tone measurement is nuanced," said Dr. Wong. "Our preliminary data at Duke revealed significant variability even within traditional racial categories—patients who self-identify similarly can exhibit a wide range of pigmentation levels. Capturing this accurately will improve our predictive models substantially."
By prospectively gathering the largest skin-tone dataset to date in critically ill populations, Dr. Wong’s study also lays the groundwork for future clinical research beyond pulse oximetry, addressing broader questions about skin tone and health care disparities.
The project includes three primary aims: developing an EHR-based machine learning model to identify hidden hypoxemia risk, enhancing the model by integrating skin tone data to improve prediction accuracy and validating both models prospectively across diverse clinical settings at Duke and Emory. This final validation step ensures robustness and generalizability, preparing the models for widespread clinical deployment.
The team’s pragmatic approach and immediate clinical applicability represent a shift toward addressing algorithmic biases and inequities using data-driven, cost-effective methods rather than relying solely on future technological advancements.
"Clinicians need solutions they can use now," Dr. Wong said. "Our models use resources hospitals already have—existing pulse oximeters, routine labs, and standard EHR data—to make immediate improvements in patient care."
Dr. Wong views his work within the broader context of advancing equitable care delivery through practical interventions.
"This research isn’t about waiting decades for perfect technology," Dr. Wong emphasized. "It’s about making thoughtful, immediate improvements using tools already at hand. In critical care, even small improvements can significantly impact patient outcomes."