
The use of sedating central nervous system (CNS)-acting medications for pain, sleep, anxiety and mood disturbances — particularly among older adults — has doubled in the past decade, creating a polypharmacy syndrome among patients that concurrently use three or more of these medications, which can adversely affect their health.
CNS-active polypharmacy in older people may increase risks for physical and cognitive impairment, and medication-related falls that result in serious injuries and hospitalization, and it presents further challenges to providers managing patients on multiple medications.
“This trend is only going to continue to grow as we see more older adults in our population,” said Duke-trained geriatrician, Dr. Juliessa Pavon, whose research program leverages informatics and artificial intelligence (AI) to improve medication safety and functional outcomes in older adults.
Building a Clinical Decision Support Model
Dr. Pavon is the principal investigator on a new $2.8 million, five-year National Institutes of Health Research Grant (R01) to help clinicians and older adults make safer, data-driven decisions about stopping medications.
Using the power of AI and millions of electronic health records, she is building a machine learning model that recommends the optimal CNS-acting medication to select for deprescribing — the systematic process of tapering or stopping higher risk medications — based on a patient’s unique clinical characteristics.
“Our ultimate goal is to develop a user-friendly clinical interface that presents both the benefits and potential risks of deprescribing medication classes,” said Pavon, who is thrilled at the prospect of using the power of AI to protect a vulnerable, older adult patient population. “Integration into clinical practice relies on trustworthy AI and transparency about how the models make decisions.”
The study is unique in its close focus on making AI and machine learning trustworthy and understandable to patients and providers, said Irina Mokrova, associate director of the Duke University School of Medicine Office of Research Development.
Data-Driven Decision Making
“Juliessa's genius is taking all this big data, all the evidence we have for each patient in the system, feeding it into the AI and letting AI make recommendations so that doctors have what is essentially a risk-assessment gauge that is data driven,” she said. “With all of the talk about the doom and gloom of AI, this is a great example of AI being used as a protective tool.”
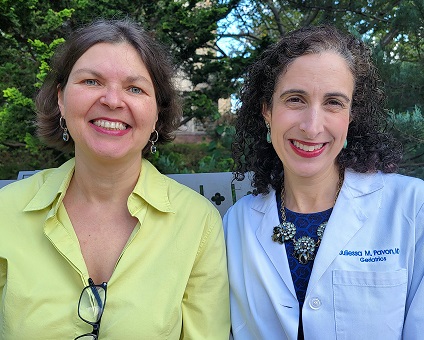
The grant also involves investigators from Vanderbilt University Medical Center and the Medical University of South Carolina. Under Dr. Pavon, the team will use PCORnet multi-site electronic health record data, linked to Medicare dispensing and claims, and causal inference methods to sort out the effects of medication discontinuation on patient outcomes from other influencing factors.
A series of design thinking group sessions involving clinician and patient end-users at each participating site will be engaged early on in the construction phase of a prototype clinical decision support tool to help develop trust in machine learning-based tools.
To ensure transparency in how the model works, explainable techniques will be used to help clinicians and patients understand how the model makes its predictions. Supervised machine learning algorithms will be trained to predict the probability of benefits or harm from deprescribing for each medication class, by integrating patient-specific characteristics. Unsupervised clustering techniques will be used to identify patterns in medication discontinuation or outcomes for subgroups of patients.
Combined, the grant will develop AI models that use patient’s individual clinical characteristics to accurately predict which medications are most likely to provide benefit or harm when deprescribed, for outcomes such as fewer falls, slower dementia changes, reduced hospitalizations, and less risk of rebound symptoms.
CNS Medication Burden
Deprescribing holds great promise to reduce the burden of CNS medication and improve the well-being of older adults — especially when personalized and data-driven support is available — but it may also exacerbate underlying symptoms for which the medications were originally prescribed. So, clinicians and patients often struggle to determine which medications to deprescribe.
Medication prescribing cascades can contribute to medication overburden when one drug leads to another then another, creating cumulative side effects that potentially start to alter what Dr. Pavon calls “the brain landscape.”
Existing guidelines do not take into consideration the complex interplay of multiple medications and individual patient’s unique clinical characteristics, which are necessary for individualized, patient-centered care. So, individualized deprescribing solutions that account for these complexities are essential, Dr. Pavon said.
The study includes collaborators from the Duke University Division of Geriatrics and Palliative Care, Drs. Nicki Hastings and Cathleen Colón-Emeric, and colleagues from the departments of Biostatistics and Bioinformatics, and Population Health.
The R01 award is an extension of Dr. Pavon’s expertise in studying AI translation from model development to deployment, employing AI predictive modeling for older adult care, and translating the efforts into clinical decisions. Her program of research is nationally recognized within the Geriatrics community, and integrates work across the Division of Geriatrics, Aging Center, Pepper Center, and Duke AI Health.
Her early research on optimizing high-risk medication use in older adults revealed that use of certain CNS-active medications during hospitalization were linked to increased readmissions. Dr. Pavon and her team further demonstrated that CNS-active medication deprescribing around hospitalization is minimal, and clinician and patients reported a desire for automated decision support tools to facilitate deprescribing.
She has also led projects aimed at improving medication safety for older adults vulnerable to falls through the Durham VA Health Care System and the Duke University Health System.